The Viability of Space Payload Transportation
In the dynamic realm of space exploration and commercial viability, a critical question looms: Is the substantial initial investment in high-complexity systems justified for businesses? This inquiry intersects with the risk and uncertainty inherent in creating reusable space vehicles, prompting a meticulous evaluation of cost-benefit dynamics.
The space industry is witnessing rapid development, with commercial launchers offering rides to orbit for various payloads. While some focus on small, expendable rockets like Astra and Rocketlab, others, like SpaceX, pursue ambitious fully reusable spacecraft. Current trends show a surge in payload traffic to orbit driven by commercial needs, alongside a gradual decline in the cost-to-orbit trajectory. However, historical lessons, such as the challenges faced by the Space Transportation System (STS), underscore the complexities of achieving semi-reusability.
Looking ahead, proposals for fully reusable systems by companies like Relativity Space and SpaceX signal a potential paradigm shift. The allure of fully reusable "heavy lift" rockets holds promise, with emerging sectors such as earth imaging and satellite servicing driving demand for LEO/GEO launches. As the industry navigates these shifts, the quest for efficiency and sustainability takes center stage, shaping the trajectory of space exploration and commerce.
Related Works
Looking back at studies like "The Space Shuttle Decision: NASA’s Search for a Reusable Space Vehicle" and "Economic Model of Reusable vs. Expendable Launch Vehicles" gives us a solid foundation for understanding how to build cost-effective rockets ready for space travel. Exploring space isn't just about overcoming physical barriers—it's also about navigating financial ones.
Economic analysis becomes crucial in this endeavor. By tackling uncertainties with a "probabilistic" mindset, as discussed in "An Overview of Methods to Evaluate Uncertainty of Deterministic Models in Decision Support," and embracing flexibility in design, as highlighted in "Flexibility in System Design and Implications for Aerospace Systems," we're better equipped to tackle the challenges of building launch systems. Now, fast forward to today, where US-based commercial launch providers are gearing up to sling satellites into Low Earth Orbit (LEO) and Geostationary Orbit (GEO), capitalizing on a booming demand for space cargo.
A pragmatic SWOT analysis helps us size up the industry, pinpointing strengths, weaknesses, opportunities, and threats. And when it comes to picking the right rocket for the job, we've got options: go expendable, semi-reusable, or aim for fully reusable vehicles like the Starship. These alternatives offer different price tags, challenges, and potential payoffs. As we strive for innovation and affordability in equal measure, it's clear: each launch is a step toward unlocking the mysteries of the cosmos.
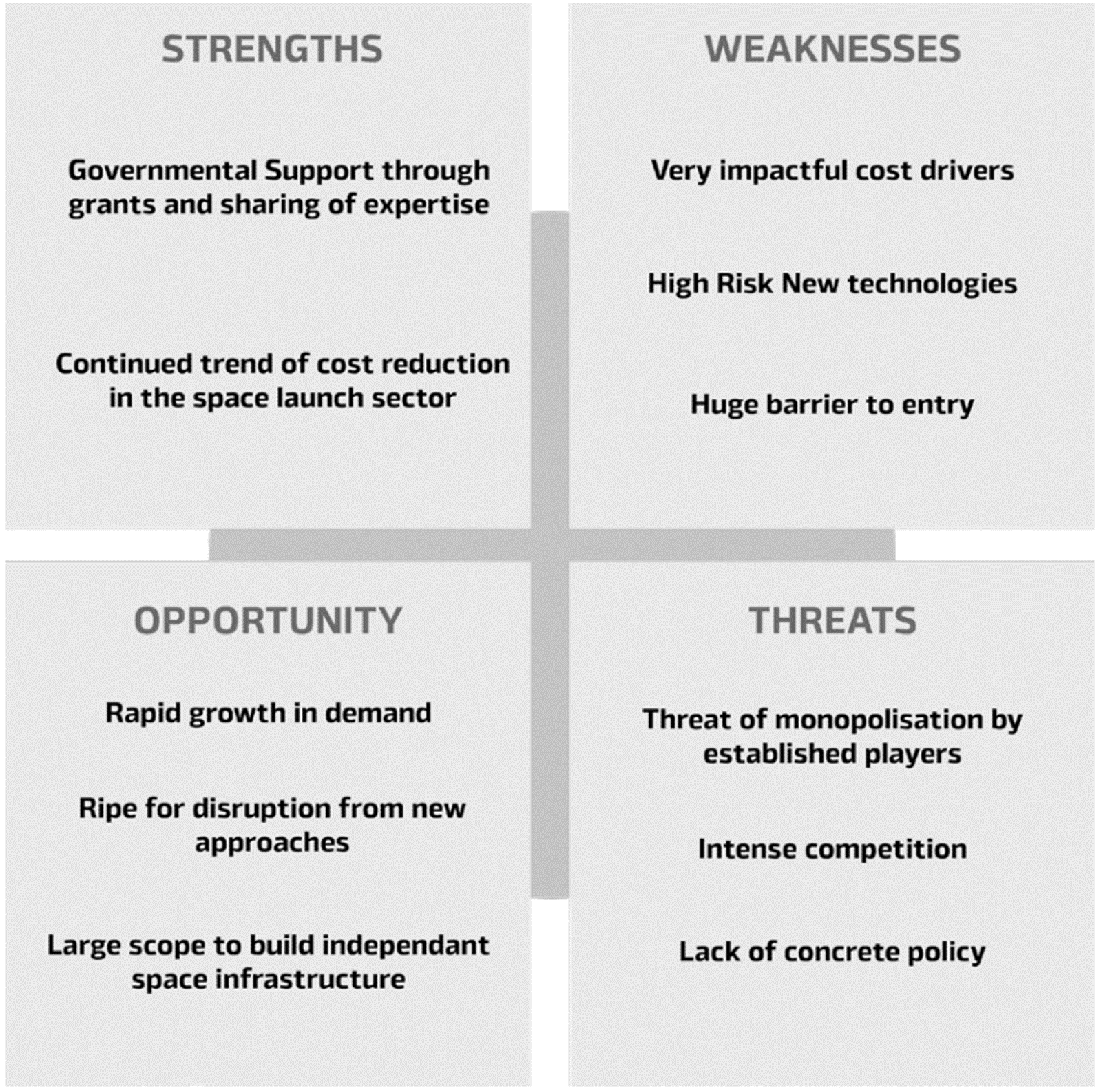
Methodology
Preliminary Market Analysis
The analysis considers costs, compound annual growth rate (CAGR) of demand, Minimum Acceptable Rate of Return (MARR), and delivery destinations for three SpaceX rockets: Falcon 1, Falcon 9, and Starship-Superheavy. Costs vary based on reusability and payload capacity. CAGR of demand for space-launch is conservatively estimated at 5.3%. MARR is set at 20% due to high risk. Revenue primarily comes from delivering objects to Low Earth Orbit (LEO) and Geostationary Orbit (GEO), with other potential revenue streams being marginal.
Evaluation Approaches and DCF
The deterministic business case involves initial capital expenditures (CAPEX) for land, research and development (R&D), and factory construction, treated as setup costs at present value within the model, with a straightforward conversion between present and annual worth. During R&D and construction, no launches take place. Operating costs include expenses for new ships, launch operations, and refurbishment, assuming a one-year construction cycle for new ships based on demand and/or lifecycle considerations, and following refurbishment cycles per ship, considering their reusability. Salvage value (SV) is calculated as a fraction of unrealized flights within the lifecycle of all operating ships, scaled by production cost, using a linear depreciation model, and discounted by the Minimum Acceptable Rate of Return (MARR). Cash flow is evaluated by deducting fixed and operating costs from revenues generated annually, with Discounted Cash Flow (DCF) obtained by discounting each value by the MARR.
Uncertainty Characterism and Modelling
Sources of uncertainty are consolidated into a single uncertainty model for alternative comparative analysis. Firstly, Year 1 demand uncertainty, given the short horizon, is expected to be minor and not sensitive to noise distribution type, addressed by multiplying the projected demand by a randomized factor. Secondly, uncertainty in the 24-year demand projection, with an expectation of upside surprises due to industry growth, is modelled using a right-skewed triangular distribution, with the average determined by the deterministic projection. Thirdly, growth rate uncertainty, reflecting fluctuations in demand due to economic conditions, etc., is addressed by a uniform distribution of deviation from the growth rate.
Destination uncertainty, accounting for fluctuations in demand composition, is treated as separable from overall demand uncertainty, modelled as noise in the ratio of demand between LEO/GEO destinations with an independent uniform random distribution. Lastly, R&D time horizon uncertainty, acknowledging variations in research into unknowns, is modelled with a binomial distribution centered around the deterministic R&D duration, generating binomial random numbers with a deviation of ±1 year from the projected horizon.
Flexibility
Since the main uncertainty is related to demand, the flexibility study will be centred around satisfying the demand dynamically in order to increase NPV. This can be achieved by building new ships on the basis of the most recent known demand evaluation. The approach can work differently for alternative scenarios, hence specific applications are described separately.